Sebastian Wetzel
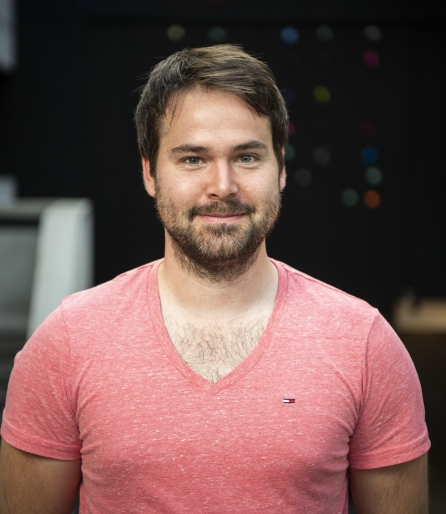
Research Interests
Theoretical Physics / Machine Learning intersection
Recent Publications
- Toward Orbital-Free Density Functional Theory with Small Data Sets and Deep Learning K Ryczko, SJ Wetzel, RG Melko, I Tamblyn Journal of Chemical Theory and Computation 2022, 18, 2, 1122-1128
- Logic Guided Genetic Algorithms D Ashok, J Scott, SJ Wetzel, M Panju, V Ganesh Proceedings of the AAAI Conference on Artificial Intelligence 35 (18)
- Discovering Symmetry Invariants and Conserved Quantities by Interpreting Siamese Neural Networks, Sebastian J. Wetzel, Roger G. Melko, Joseph Scott, Maysum Panju, Vijay Ganesh Phys. Rev. Research 2, 033499
- Unsupervised Learning of Rydberg Atom Array Phase Diagram with Siamese Neural Networks Zakaria Patel, Ejaaz Merali, Sebastian J Wetzel arXiv preprint arXiv: 2205.04051
- Twin Neural Network Regression is a Semi-Supervised Regression Algorithm SJ Wetzel, RG Melko, I Tamblyn arXiv preprint arXiv: 2106.06124
- Twin Neural Network Regression SJ Wetzel, K Ryczko, RG Melko, I Tamblyn arXiv preprint arXiv: 2012.14873
- Modern applications of machine learning in quantum sciences, Phase Classification Anna Dawid, Julian Arnold, Borja Requena, Alexander Gresch, Marcin Plodzien, Kaelan Donatella, Kim Nicoli, Paolo Stornati, Rouven Koch, Miriam Büttner, Robert Okula, Gorka Muñoz-Gil, Rodrigo A Vargas-Hernández, Alba Cervera-Lierta, Juan Carrasquilla, Vedran Dunjko, Marylou Gabrié, Patrick Huembeli, Evert van Nieuwenburg, Filippo Vicentini, Lei Wang, Sebastian J Wetzel, Giuseppe Carleo, Eliska Greplová, Roman Krems, Florian Marquardt, Michal Tomza, Maciej Lewenstein, Alexandre Dauphin arXiv preprint arXiv: 2204.04198
Seminars
- Interpreting artificial neural networks in the context of theoretical physics, Machine Learning for High Energy Physics, on and off the Lattice, Trento
- Challenging Traditional Beliefs in Machine Learning, Summer school: Machine Learning in Quantum Physics and Chemistry, Warsaw
- Interpreting artificial neural networks in the context of theoretical physics, Artificial Scientific Discovery, University of Erlangen-Nuremberg
- Interpreting artificial neural networks in the context of theoretical physics, Mila, Quebec AI Institute
- Interpreting artificial neural networks in the context of theoretical physics, CAP Virtual Congress
- Siamese Neural Networks Learn Symmetry Invariants and Conserved Quantities, ML Club, CEA Saclay, Johns Hopkins, IAC, Paris Observatory, UC Santa Cruz
- Can We Trust Phase Diagrams Produced by Artificial Neural Networks?, First French-German Meeting in Physics, Mathematics and Artificial Intelligence Theory, Paris
- Machine Learning Phase Diagrams of the Ising Model and SU(2) Lattice Gauge Theory, Waterloo
- Machine Learning of Explicit Order Parameters: From the Ising Model to SU(2) Lattice Gauge Theory
- Twin Neural Network Regression
- Siamese Neural Networks, 24th march 2020
- PIRSA:19040090, Can we trust phase diagrams produced by artificial neural networks?, 2019-04-11, Machine Learning Initiative